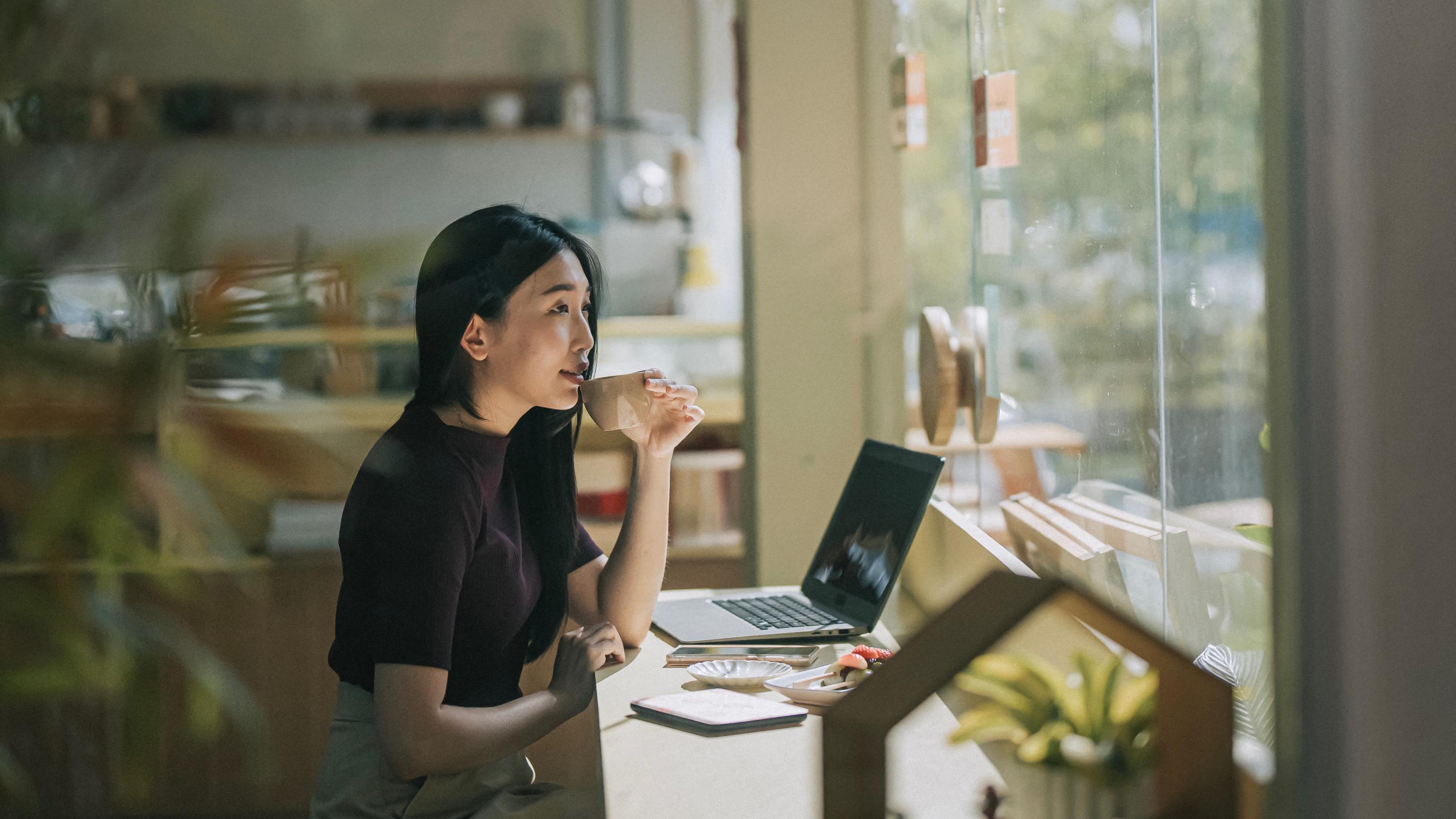
Creating a Data-First Culture: The Essential Foundation for AI Success
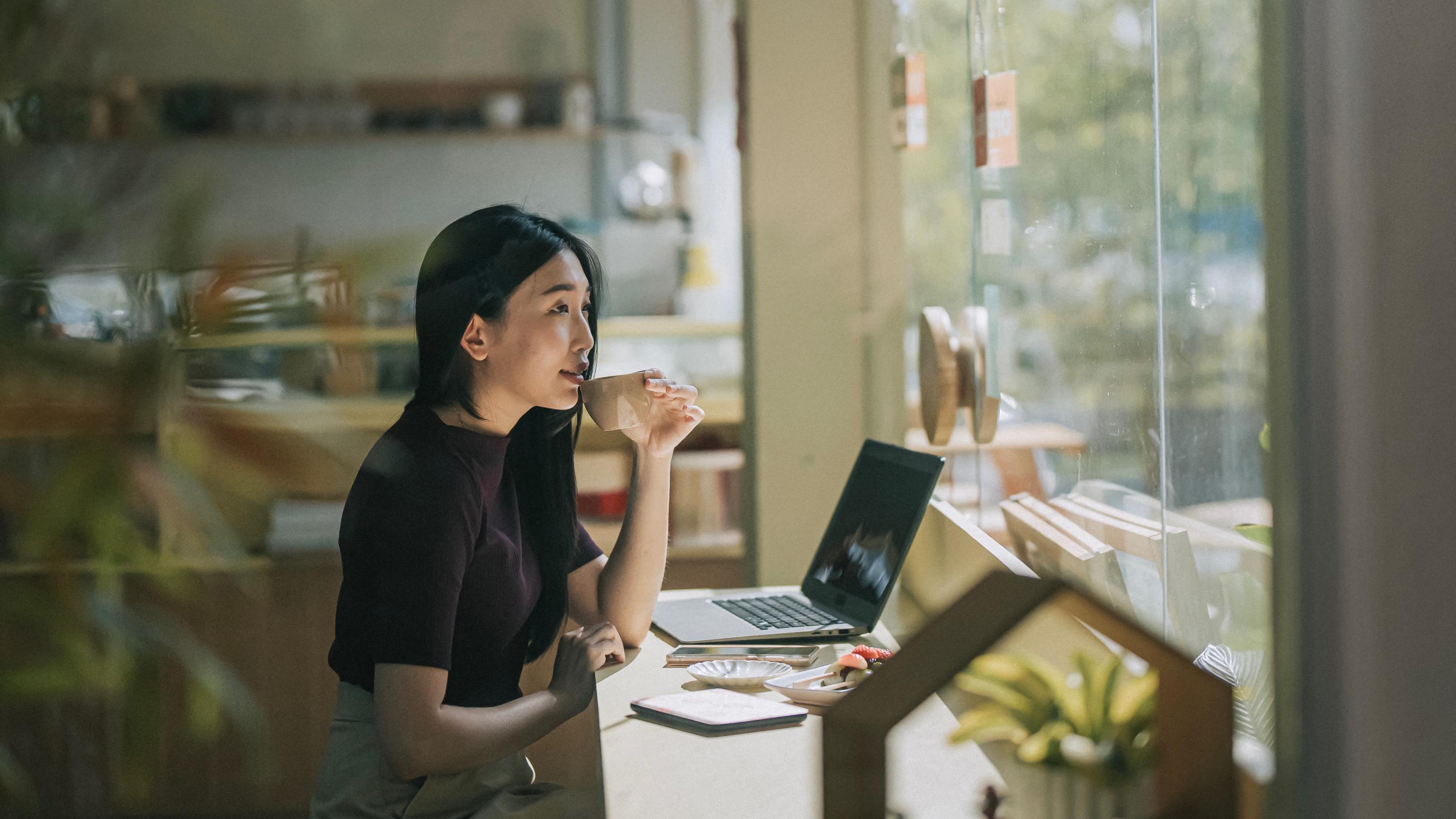
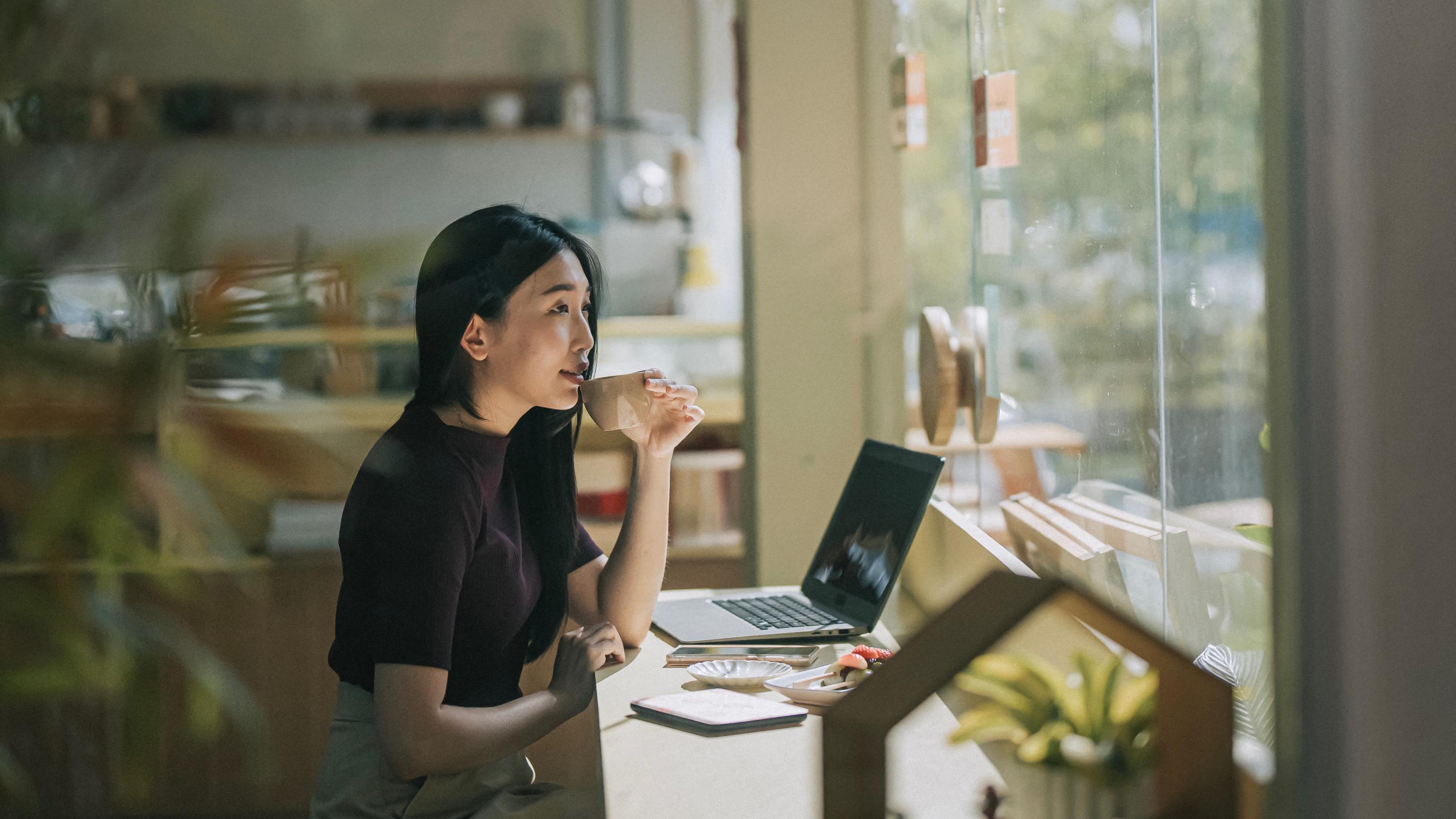
Introduction
Like many of you, over the last couple of years I've been wrapping my head around the ever-changing landscape of Artificial Intelligence. What is a model? How do you train it? What about an agent? Large Action Model? How is AI different from Machine Learning? The list goes on. One could spend the better part of a week just getting to a point where they know the right questions to ask, only to find all of your questions outdated because the field has advanced in the time it took you to catch up. This is daunting for any organization looking to understand what AI can do for them. Where do you even start?
In thinking about this, it is critical to remember a trivial but often overlooked fact about Artificial Intelligence. Everything an AI can do is rooted in the data you feed it. Functionally, if you give an agent the wrong data, or mislead it about how data should be used, it will confidently deliver inaccurate or out of context information. When we don’t have a handle on where our data lives, and governance in place to ensure its accuracy, we drastically increase the chances of giving our agents bad data and limiting their capabilities. When we take this into account, it becomes very clear where we should start: the data!
Any organization looking to leverage AI to its fullest must understand the importance of preparation before adoption. While technical investment is necessary, the bulk of the work lies in understanding what factors drive your organization's KPIs and identifying areas where AI can realistically accelerate results. This clarity reveals what data you need, how to transform it, and how your agents should use it. It's through this tactical approach to data and AI that organizations begin their transition to becoming truly data-first.
What does it mean to be Data-First?
Being data-first is fundamentally a mindset shift. It means seeing data as more than just information in a database, but as the foundation for AI, decision making, and business strategy. Organizations looking to transition to a data-first approach must ask themselves: What information are we missing? What don't we know about our customers, clients, or internal operations? And how do we quantify that? Understanding the key factors in your business's success and how to capture them is a prerequisite for making this transition.
Data-first organizations embed the right data at the right time into their decision-making workflows. The concept is actually quite intuitive. Would you ever give a speech to an audience without first doing your research? Every organization is data-first in some ways, but what's key is having a comprehensive and intentional strategy for how you harness data to tell stories that guide you in specific situations.
With advances in generative AI, we've unlocked powerful new ways to help you leverage your organization's data. Together, we can now process documents, audio files, and other forms of unstructured data at scale to extract meaningful insights that were previously inaccessible. Where you once had only numbers, we can now provide the broader context behind those figures. AI agents can connect these disparate pieces, giving you a comprehensive view of your business landscape and helping you make more informed decisions.
While AI's storytelling capabilities are impressive, they deliver the most value when deployed with clear intentions. We recommend developing AI agents tailored to your specific use cases, with your data structured to support these exact needs. Reaching this point can be tricky, but we can work with you to identify precisely which questions your business needs to answer, ensuring your AI investments deliver tangible results.
Asking The Right Questions
The data cleaning process requires us to have a clear vision of how we need to transform and aggregate our data to fulfill specific needs. While AI can help with this process, at its core, this is fundamentally a human challenge. Landing on the right questions requires a collaborative effort from the entire business to identify what truly drives the organization forward. These questions exist in layers—some are more relevant at the departmental level, while others are critical at the organizational level. Once a solid data foundation is established, these questions become key in determining how each department taps into this collective well of knowledge.
Asking the right questions leads to storing the right data, which in turn leads to clearer instructions for your AI agents. This approach not only increases the quality of AI outputs but can also ensure cost effectiveness and optimized performance by decreasing the amount of irrelevant data the agent must process. Of course, this is easier said than done. Too often, we find ourselves working in silos, where cross-functional collaboration becomes increasingly challenging as organizations grow larger and more complex.
It's precisely because of these challenges that the shift to becoming data-first works best when it’s a collaborative effort that involves multiple groups and/or departments in your organization. Investment in governance planning and belief in the benefits of data hygiene must permeate all levels of the organization. While we often focus on the technical or business aspects of this transformation, it's important to remember this is more of a culture shift than anything else.
Shifting to a data first culture
Painting a clear vision is only half the challenge in our transformation. While it can be relatively straightforward to envision how your organization could leverage its data, inspiring your people to become willing participants and champions of the necessary structures presents a more complex hurdle.
For data to play a more prominent role in your organization, leadership must be challenged to identify the key factors driving performance in their departments and empowered to question the status quo. This investment makes leveraging AI tools more effective, as you'll have already mapped where the context for business activities resides.
With proper AI implementations, employees will experience firsthand how these technologies can revolutionize their workflows. Whether it's agentic systems that automatically embed insights directly into tools like Teams, Outlook, and SharePoint, or solutions like Copilot that summarize meetings and deliver action items, users will begin to see the tangible ways AI helps them every day—and become genuinely excited about the possibilities.
When people experience these practical benefits directly in their daily work, resistance naturally diminishes, and adoption accelerates. The key is starting with high-impact, low-friction use cases that demonstrate immediate value while building toward your larger data governance vision.
Over time, this approach creates a virtuous cycle. As employees and leadership witness AI's tangible benefits, they'll naturally begin to identify new potential applications in their areas of expertise. More importantly, with a proper understanding of AI's capabilities and limitations, they'll instinctively focus on the data requirements needed to empower these new use cases. Questions like "What data would we need to make this possible?" and "How should we structure this information to maximize AI effectiveness?" become part of everyday strategic thinking.
Data First Takes Time
Keep in mind there's no shame in starting small! Consider selecting one department as a pilot program and begin by identifying what kind of data they need and exploring how to collect and maintain that data store. This "foot in the door" approach can be particularly effective in demonstrating the power of AI when connected to clean data sources, which can in turn drive internal excitement and create demand for similar implementations across other departments.
When it comes to data governance plans, you must take into account the existing company culture. Unless you're launching a new company, your organization likely has established ways of operating. When possible, it's best to meet your organization where it is currently, opting for gradual implementation of data hygiene processes rather than radical change. Stay attuned to feedback from departments and leaders about what's working and what isn't.
Remember that cultural transformation takes time. The shift to becoming truly data-first may require months or even years, depending on your organization's size and complexity. However, with consistent leadership support and a pragmatic, people-centered approach, the foundation for AI-ready data governance can be successfully established.
Conclusion
The path to becoming a data-first organization requires more than technological investment—it demands a cultural shift touching every corner of your organization. The goal isn't data collection for its own sake but creating the foundation that enables AI to deliver meaningful insights that drive business forward.
Strive to achieve some quick wins.. Focus on one area where clear, well-structured data could make an immediate impact and use those early successes to build momentum. Cultivate champions across departments who understand both the technical requirements and business benefits of this transformation.
Be patient and persistent. By taking deliberate steps toward data governance excellence today, you're positioning your organization to harness AI's full potential tomorrow. In a world where AI capabilities evolve at breathtaking speed, the organizations that will lead aren't necessarily those with the most advanced models, but those with the cleanest, most relevant data to fuel them.