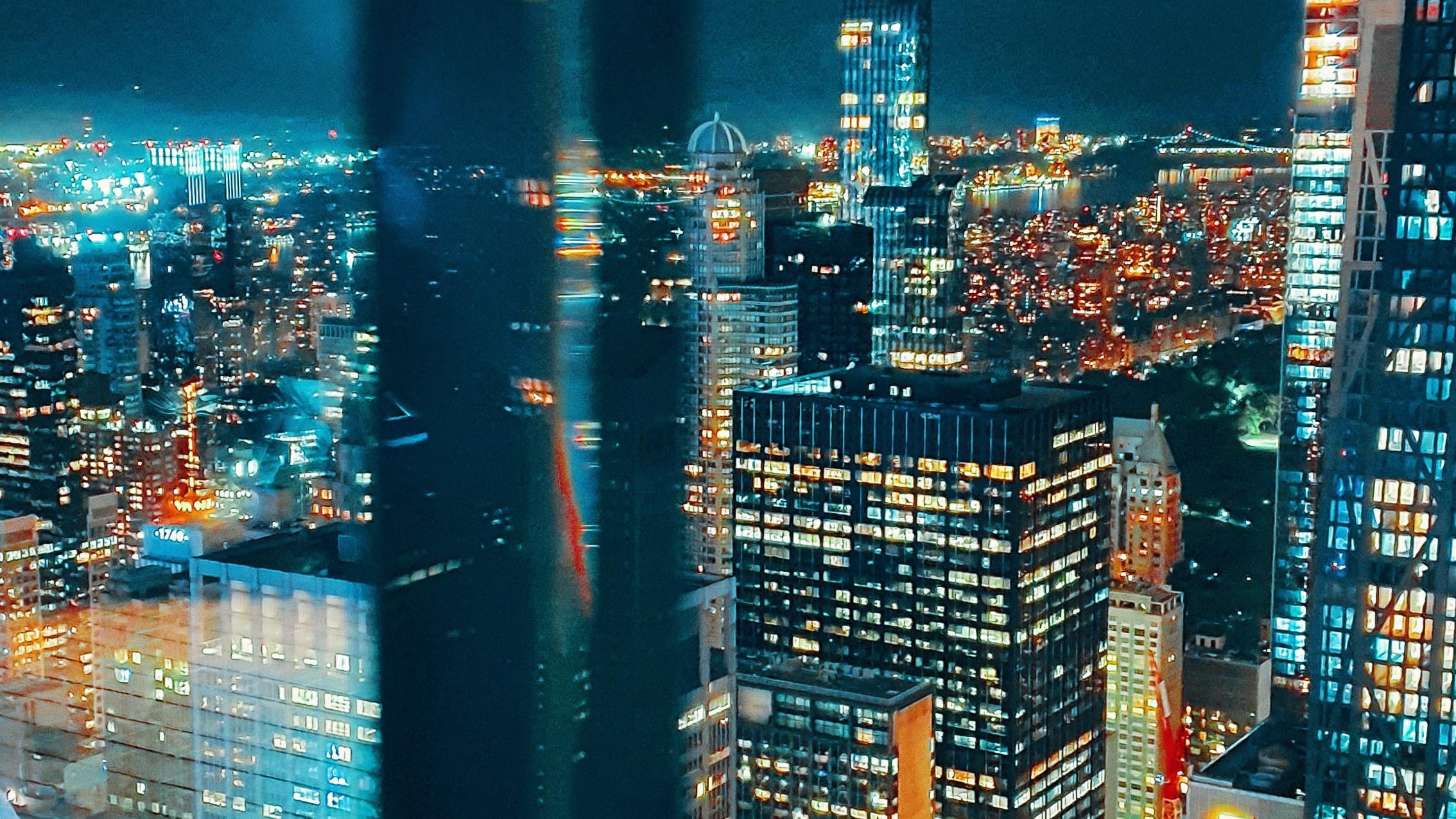
Building an AI Culture at Rightpoint: Lessons To Harness Experimentation for Practical Impact
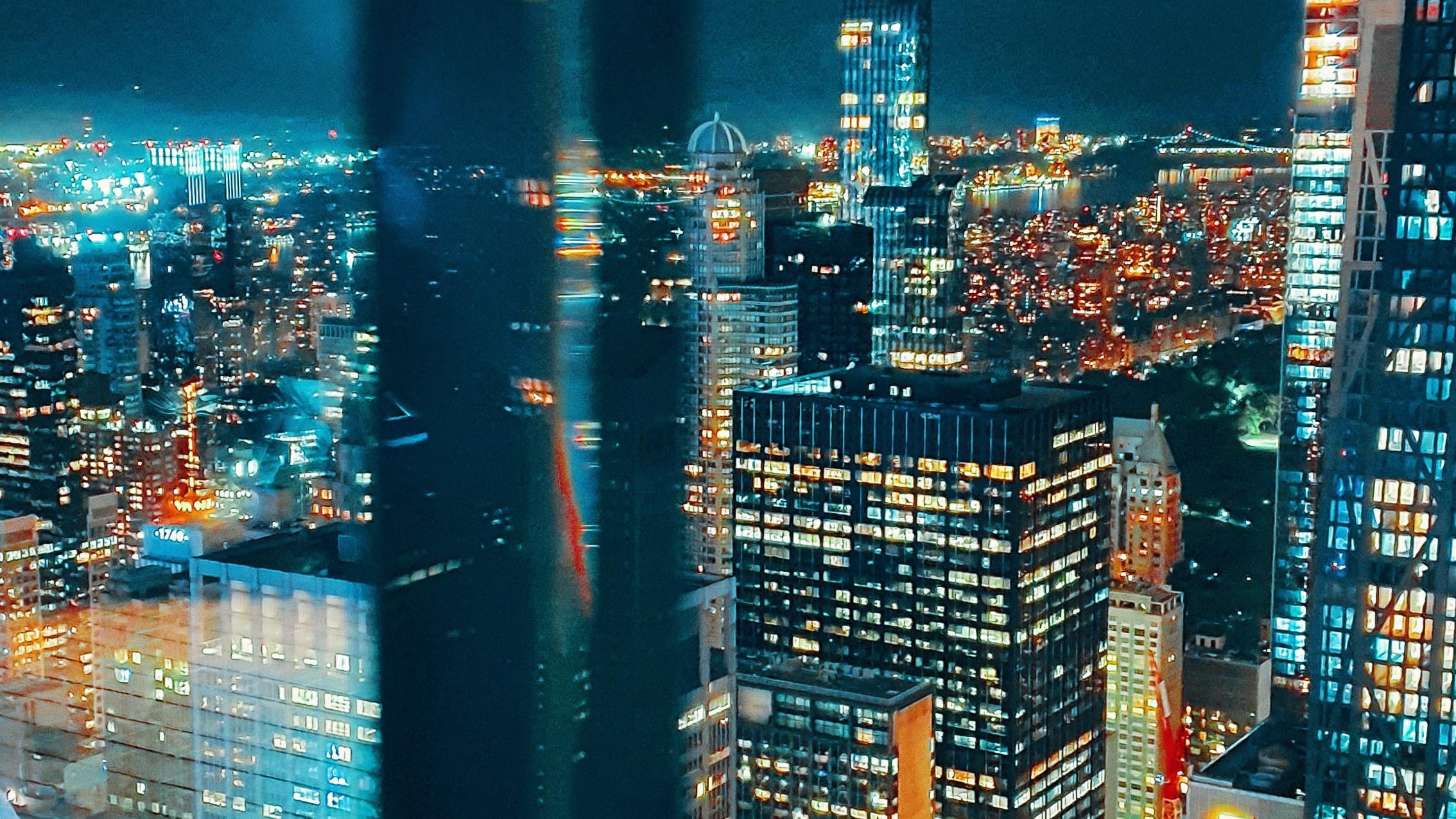
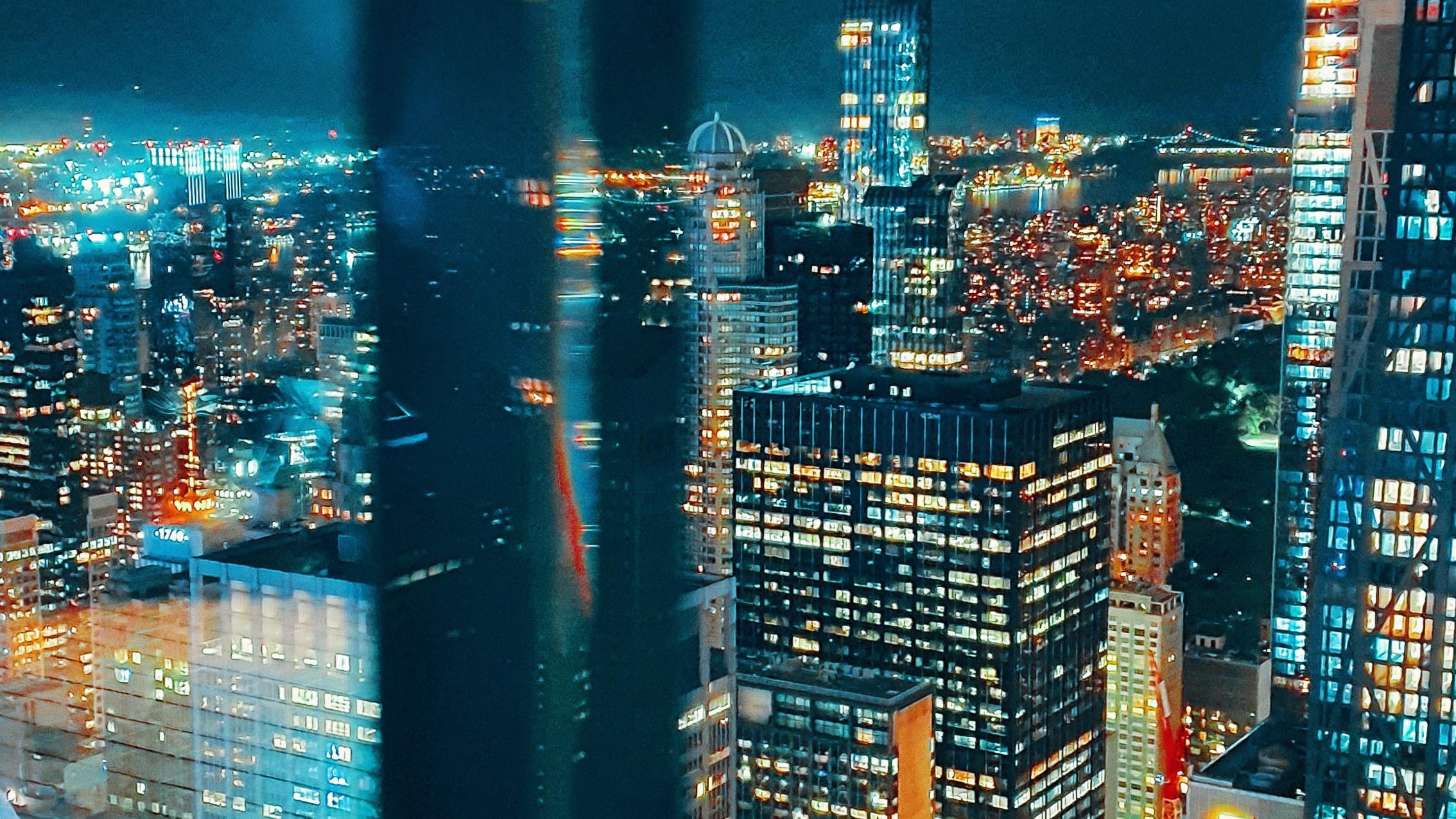
AI is everywhere, and it can be downright overwhelming. With its rapid advancement, finding a place to start can feel like trying to drink from a firehose.
Sometimes, the best way to begin using AI is to start experimenting with it. Experimentation was the main idea behind the Rightpoint AI hackathon this year. The main goal of the hack-a-thon was to develop some sort of proprietary AI tool. This allowed us to hit the road running when it came to determining how we might implement AI in some of our everyday business challenges.
One of the biggest things we learned from this experience was the value of empowering small teams to work quickly and experiment with solutions to problems that matter most to them. In this article, we will elaborate on some of these findings and discuss how small experiments can lead to impactful insights and help shape your approach to leveraging AI in your organization.
Experimentation Puts Us in the Problem-Solving Mindset and Helps Us Identify New Use Cases
Ideating can be a deeply personal experience, and sharing your ideas can be intimidating, especially if you fear the judgment of your colleagues. Creating a controlled environment in which employees feel comfortable being honest and feel supported developing solutions is crucial. Our hackathon was a call to arms, empowering groups to self-organize around organizational issues they felt passionately about. Most problems are layered, so promoting cross-disciplinary collaboration ensured that solutions considered multiple angles of an issue.
The hack-a-thon was contained to 10 hours over a week and participants were challenged with taking abstract concepts around AI and distilling them into workable solutions. The time constraints encouraged teams to adopt a “Learn by doing” mentality when experimenting with generative AI tools. This hands-on experience not only deepend participants' understanding of generative AI capabilities, but also exposed them to the tools available today. As a result, we expect to be more capable and confident when it comes to identifying use cases for generative AI and thinking through how to bring those solutions to life.
Identify Where AI Can Help and Where It Can’t
It’s important to remember that despite its convincing output, AI is not intelligent in the same way humans are. While we can interact with a model as if we are conversing with another person, AI lacks common sense, and the accuracy of its output diminishes when asked questions beyond its programmed context. AI generates content based on its context; if fed incorrect or biased information, it will produce incorrect or biased output.
With this in mind, we felt it best to get to know AI in low-risk scenarios in which we could have human intervention adjust the output of the system if necessary. A couple scenarios that we’ve identified as low-risk include:
Meeting summarization
Goal generation
FAQ chatbot with Microsoft Copilot
Document scaffolding
Marketing content generation
One hack-a-thon team decided to focus on annual goal creation for their project. The main idea is that users would fill out a bio about their work experience and focus for the year, and the system would take that user data and pair it with RP company goals and industry trends to generate relevant annual goals.
Some key things that stood out about this use case are:
It’s relatively low risk. If the system were to generate a bad goal, there is opportunity for humans to make adjustments to make the output more meaningful.
Context is available. Considering that we create new goals each year, we already have years’ worth of data to use as context for the system
There is opportunity to streamline. How we create goals is an important part of how we function as an organization. By introducing efficiency here, we stood to improve the relevance of goals company wide and do so in a fraction of the time
It can be a tool for the people. Yearly goals keep us pushing towards our broader career goals. By giving our employees a tool like this, we’re making it easier for them to feel supported in defining their career trajectory by creating specific, personalized goals.
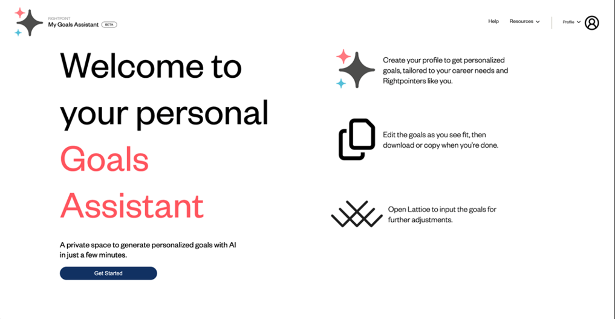
By strategically focusing AI tools such as this in low-risk situations that have the potential to boost user growth and productivity, we can increase user trust in these tools and set up a foundation for continued experimentation and development.
Fast and Frequent Feedback
AI moves quickly, so it’s vital to keep tabs on user sentiment and expectations. For our goal generation tool, we prioritized a human-centered experience, ensuring that we obtained user feedback before and after making major changes to the application. After the initial build, we interviewed users and discovered that they expected a chat-centric experience as opposed to the more static structure we originally developed. That type of feedback is crucial when building LLM-powered applications to meet the expectations of your users and will help ensure users’ investment in and continued adoption of the product.
Managing scope creep is also crucial in this stage. Our goal is to stay agile enough to quickly pivot to address incoming feedback and avoid getting bogged down in the infinite number of directions a product could go. Striking a balance between these two things can be tough but can allow design and development teams to build an offering that people will love in half the time.
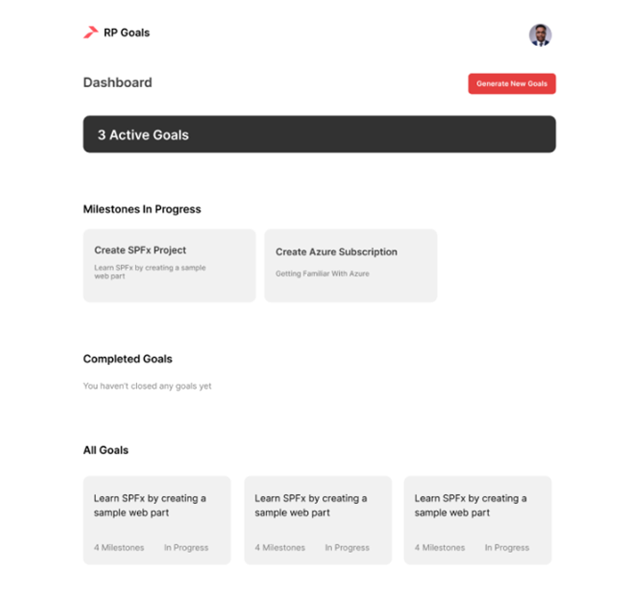
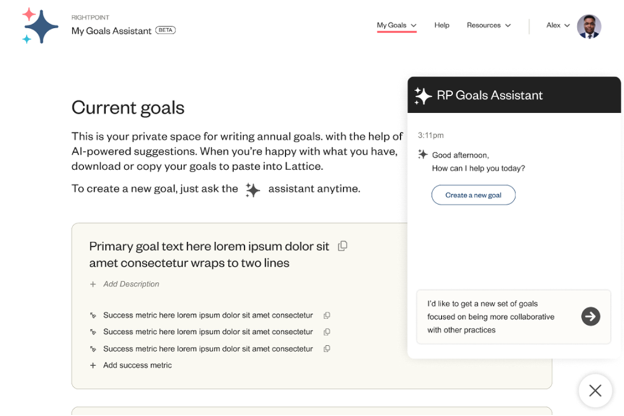
Emphasis on Organic Growth and Adoption
Driving adoption of any technology is challenging, but AI tools present unique challenges due to their ability to mimic human reasoning. Throughout time, humans’ defining trait has been our ability to reason. We are now faced with technology of our own creation that rivals (and in some cases surpasses) our own reasoning capabilities. While this naturally causes many of us to be skeptics, we do need to figure out how to trust AI as we begin to adopt it more and more.
Trust is earned, not given. When we're thinking about how to roll AI tools out to our organization, we must consider how to pair them with guidance that will enable users to confidently leverage what we build. Keeping humans first in mind as we build AI tools can help foster a sense of ownership in users that leaves them feeling supported in their work environment.
Conclusion
It’s crucial to develop your organization's relationship with AI. Identifying ways to use AI to solve small, low-risk problems within your organization allows you to build a baseline process for leveraging these tech tools. Gradually introducing AI-enabled tools can serve as a foundation for more extensive, impactful AI initiatives in the future. By starting small and fostering a culture of experimentation, you can unlock the potential of AI to drive innovation and efficiency in your organization.