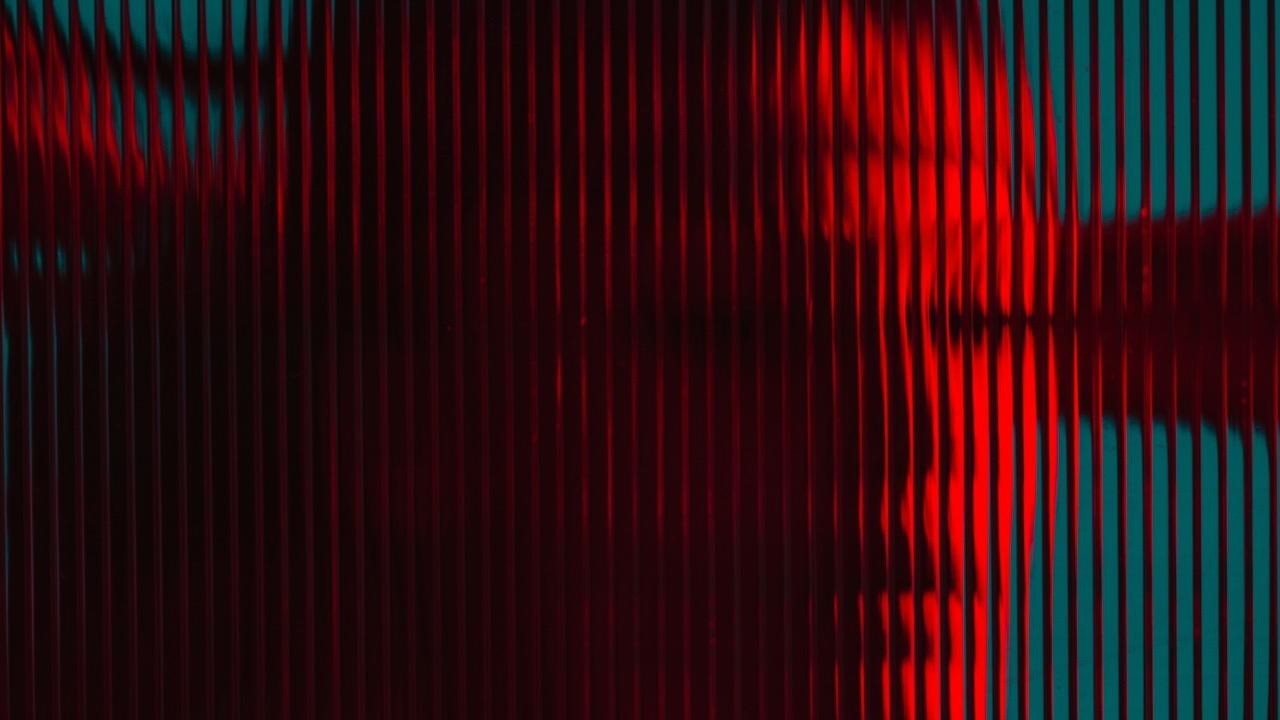
Evolving Our Approach to GenAI: Research, Use Case Identification, and Rollout Strategies
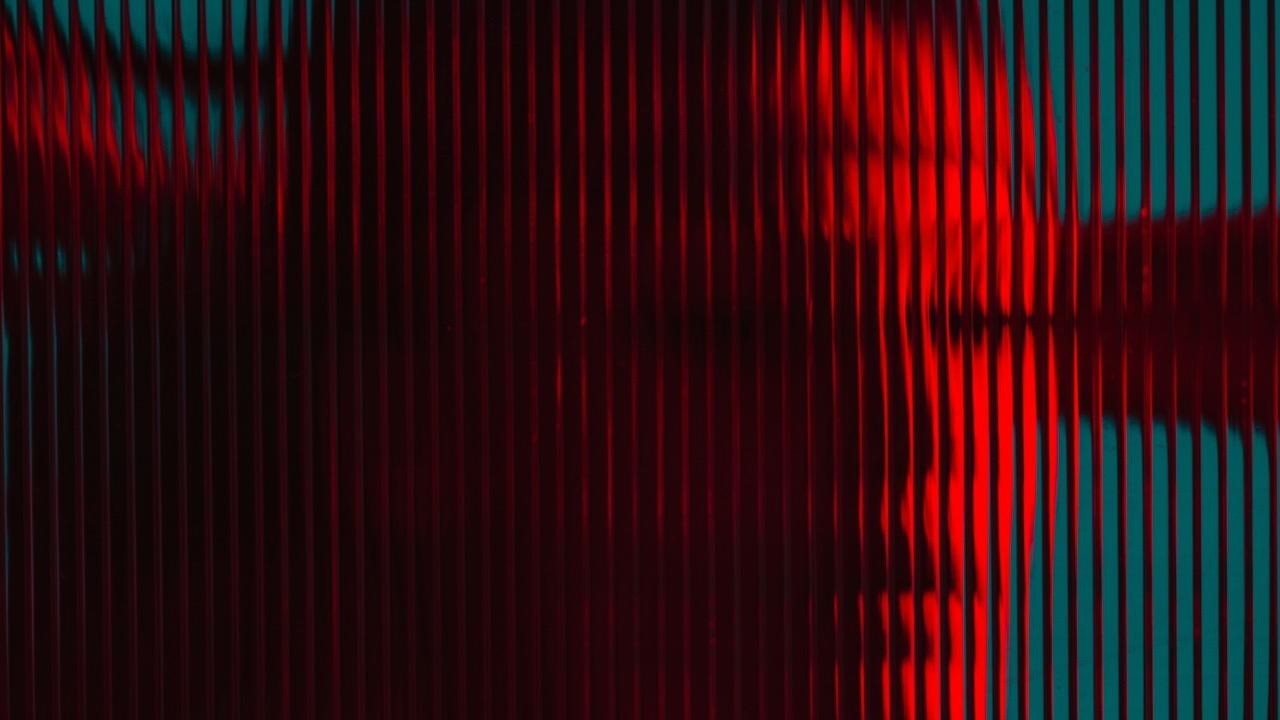
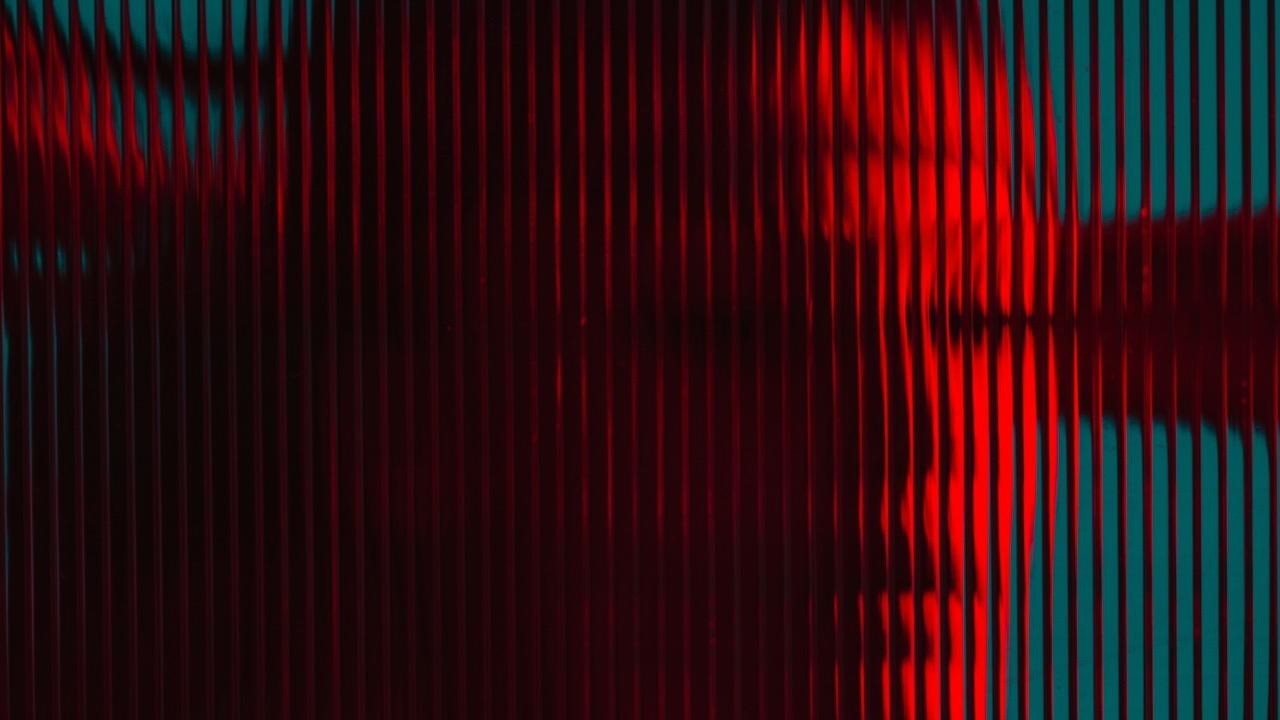
As we’ve partnered with organizations to implement Generative AI (GenAI), our thinking has evolved across three critical areas: our research approach, how we identify top use cases, and the strategies we use during rollout. Each evolution reflects the changing landscape of employee attitudes, organizational needs, and the maturity of AI technologies.
Learning 1: Research Has Become a Change Management Activity
When we first started researching GenAI adoption, employees had little knowledge or concern about how AI could impact their roles. That is no longer the case. Today, employees come to interviews with opinions, anxieties, and experiences shaped by media narratives and personal exposure.
This shift has fundamentally changed how we approach research. Instead of simply gathering insights about workflows and challenges, our research process now actively manages expectations, calms fears, and builds enthusiasm for AI’s potential. By positioning AI as a collaborative assistant—one that augments their abilities rather than threatens their job security—we ensure that employees see GenAI as a partner in their success.
Our most effective tool in these conversations is a simple yet powerful question: "Imagine you had an assistant or intern to help with your workload. What kinds of tasks or work would you want help with?"
This question not only sparks imagination but also redirects the narrative from fear to possibility. Employees begin to articulate areas where they feel overwhelmed or where AI could ease repetitive, time-consuming tasks. It’s a crucial step in creating a shared vision for how GenAI can add value to their roles.
Learning 2: Reliability Is Now the Most Important Dimension of Use Case Selection
Early in our journey, we identified use cases by focusing on value, feasibility, and potential ROI. These factors were critical to building a business case for GenAI. But as we gained more experience, we learned that reliability is just as, if not more, important—especially in the early stages of adoption.
The first interactions employees have with GenAI set the tone for their trust and willingness to engage further. One unreliable or frustrating experience can derail adoption, making users disinterested or skeptical of the technology’s potential. To combat this, we now prioritize use cases that deliver consistent, reliable outcomes.
While reliability might not always yield the flashiest use cases, it creates a foundation of trust. For example, automating email drafts or summarizing meeting notes may not feel revolutionary, but when done reliably, these tasks demonstrate the technology’s value in an accessible, low-risk way. Employees who experience early wins are more likely to experiment further and champion GenAI within their teams.
A few use cases that are low-risk and reliable to start with include:
Content Generation and Drafting: Generates drafts for emails, reports, presentations, marketing copy, job descriptions, or other written content.
Summarization: Summarizes documents, meeting notes, emails, or even data-heavy reports into concise, actionable points. Also aids in retrieving relevant information from large datasets or knowledge bases.
Information Retrieval from Other Systems: Surfaces information from other systems without having to log in. Integrations aren’t easy, but these time savers can build a lot of trust.
Idea Generation and Brainstorming: Provides suggestions, ideas, or solutions during brainstorming sessions for projects, campaigns, or problem-solving activities.
Learning 3: From Skillsets to Mindsets: Shifting the Way We Roll Out GenAI
In earlier projects, our focus was on helping employees learn the mechanics of using GenAI tools—how to craft specific prompts and follow detailed workflows. We spent significant time providing instructions, templates, and best practices for how to get the most out of AI.
However, we quickly realized that this approach had limitations. Employees often felt boxed in by overly prescriptive guidance and struggled to adapt when outcomes didn’t match expectations. As a result, we’ve shifted our emphasis to fostering a GenAI mindset instead of just building skillsets.
This mindset is about embracing experimentation and iteration. Employees need to understand that GenAI tools aren’t perfect but can be immensely valuable when used collaboratively. We encourage users to think of their interactions with GenAI as a conversation—starting with an 80% correct answer, refining it through dialogue with the tool, and then adding human judgment for the final 20%.
By shifting expectations from “perfect results” to “progressive refinement,” employees become more resilient and open to exploring GenAI’s capabilities. This mindset helps teams build confidence, embrace flexibility, and see mistakes as learning opportunities.
Learning 4: Peer-to-Peer Knowledge Sharing Is the Secret to Adoption
One of our biggest learnings has been the power of organic, peer-based knowledge sharing. While formal training programs provide a strong foundation, real adoption happens when employees see their colleagues using GenAI in ways that directly resonate with their own challenges.
For example, when a team leader casually mentions how they use GenAI to draft proposals or analyze data faster, it sparks curiosity and lowers the barrier to entry for others. Employees feel more confident experimenting when they see trusted peers finding value in the tools.
Research from Harvard Business Review reinforces this point: managers’ behavior is critical to team adoption. When leaders openly embrace GenAI and model its use, they set a tone of acceptance and innovation that trickles down through their teams. Similarly, when organizations identify “change champions” and create forums for knowledge-sharing—such as lunch-and-learns, team meetings, or dedicated Slack channels—the ripple effect accelerates adoption across departments. Finding change champions is highly dependent on your organization. Some organizations have dedicated pods of early adopters who routinely try out and evangelize new technology, some organizations have informal networks of go-to people who organically are ahead on the technology curve, and other organizations will require a more structured process for seeking out these champions (more to come on this in a future post!)
This peer-driven approach also aligns with a broader truth about workplace change: employees trust and learn best from their colleagues. By fostering an environment of shared learning and experimentation, organizations can create a culture where GenAI adoption becomes a natural, collective process.
Learning 5: Rethinking Impact: Why Measuring GenAI Success Is More Art Than Science
One of the biggest challenges we’ve faced is quantifying the true impact of GenAI adoption. While time savings are often the most tangible metric, they don’t always tell the full story—and even those numbers can be misleading.
For example, self-reported data from employees is often skewed. Some may overestimate the time saved to justify their enthusiasm for the tool, while others may underreport usage because they fear scrutiny or have yet to fully embrace the technology. Additionally, frequency of use doesn’t always correlate with impact.
Consider this: if someone uses GenAI once for three hours and saves a week of work, is that less impactful than someone who uses it daily to save 30 minutes? These nuances make it difficult to rely solely on usage data or time savings to measure success.
Furthermore, focusing only on time misses a critical dimension: quality of work. While GenAI may expedite processes, its real value often lies in improving the work itself—delivering deeper insights, crafting more polished communications, or enabling creative breakthroughs. However, measuring quality improvement is far less straightforward.
To address these challenges, new dashboards and analytics tools are emerging to provide better visibility into how GenAI is used. These tools can track patterns, such as what tasks employees are automating or how often they iterate on AI outputs. While promising, they still only capture part of the picture.
Perhaps the most important shift we’ve observed is in leadership’s evolving mindset. Leaders are beginning to treat GenAI less as an ROI-driven initiative and more as a basic requirement for staying competitive in the marketplace. This shift allows organizations to focus less on perfect metrics and more on fostering the behaviors and cultures that make GenAI adoption successful.
Ultimately, while measurement remains a work in progress, we’re learning that the true impact of GenAI is often felt in ways that can’t be neatly quantified—through better outcomes, stronger team collaboration, and the ability to stay ahead in a rapidly evolving business landscape.
Looking Ahead: A Foundation for Sustainable GenAI Adoption
As our approach has evolved, one thing remains constant: successful GenAI adoption is about more than just tools. It’s about understanding people, managing change, and creating an environment where experimentation, learning, and collaboration can thrive.
Our evolving practices ensure that GenAI isn’t just introduced—it’s embraced as a transformative force for employees and organizations alike. By focusing on research that manages change, prioritizing reliable use cases, and enabling peer-driven adoption, we’re helping organizations unlock GenAI’s full potential in ways that are practical, sustainable, and meaningful.