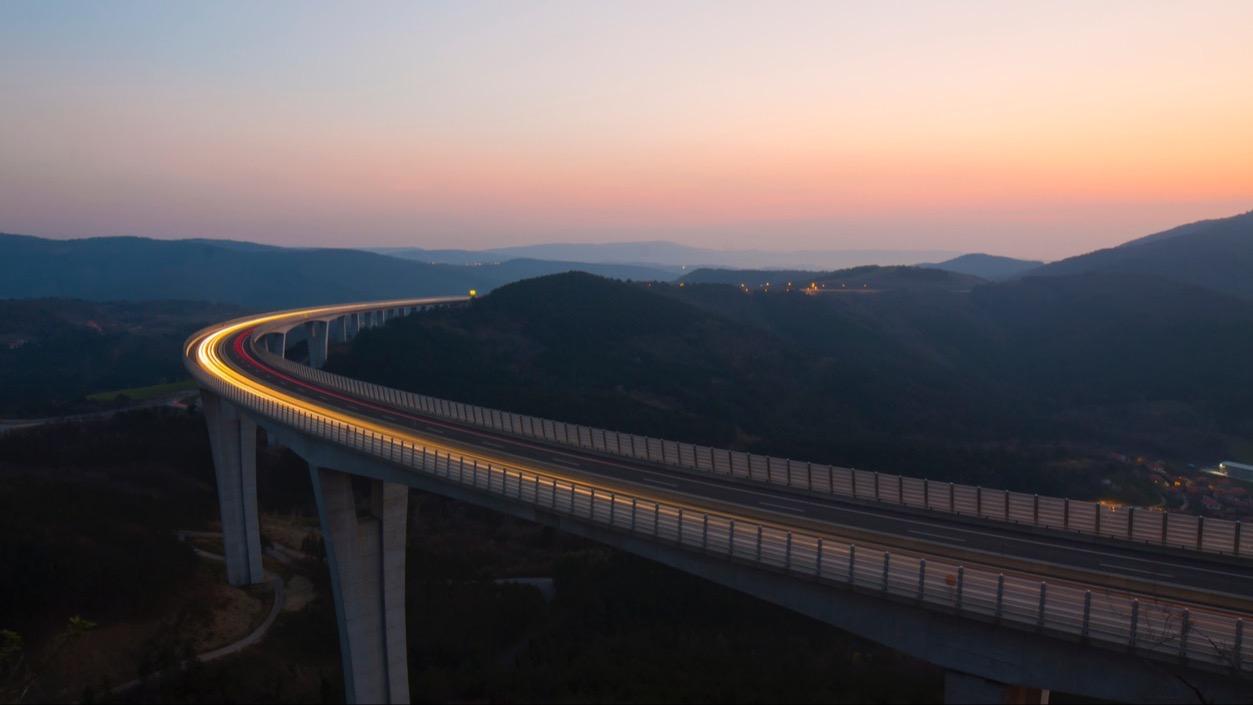
Customer Segmentation Models: Enhancing Targeted Marketing Strategies
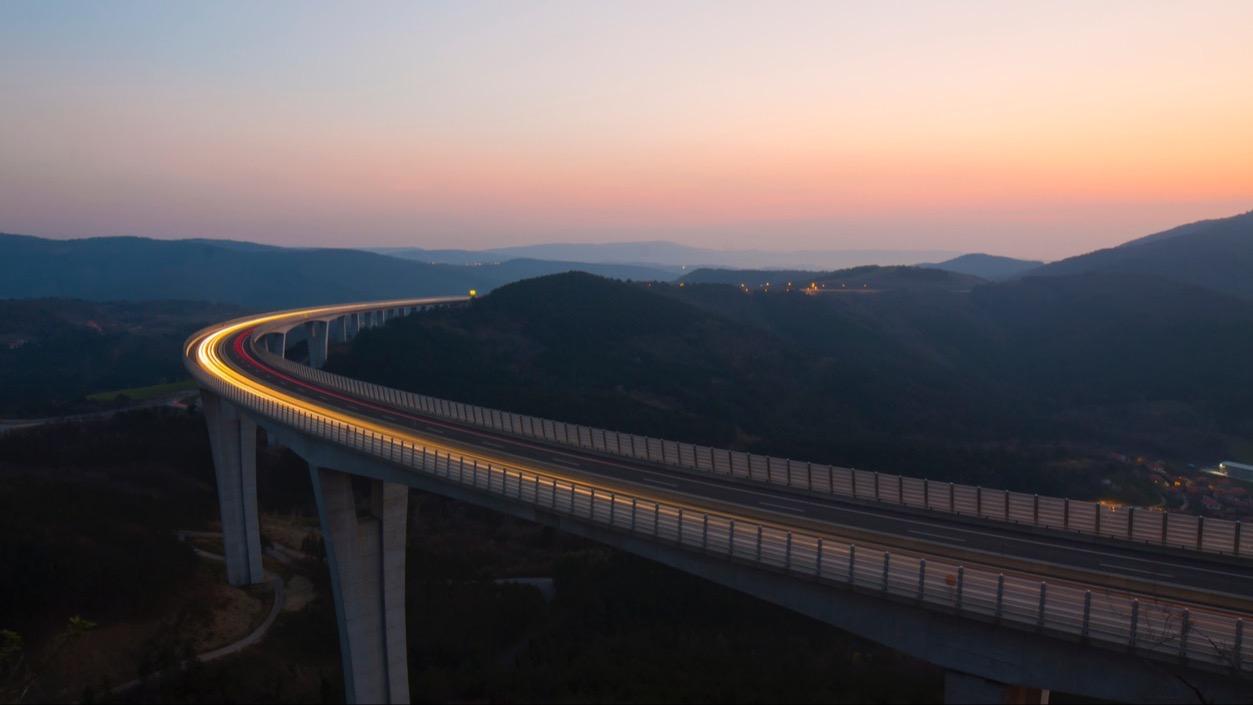
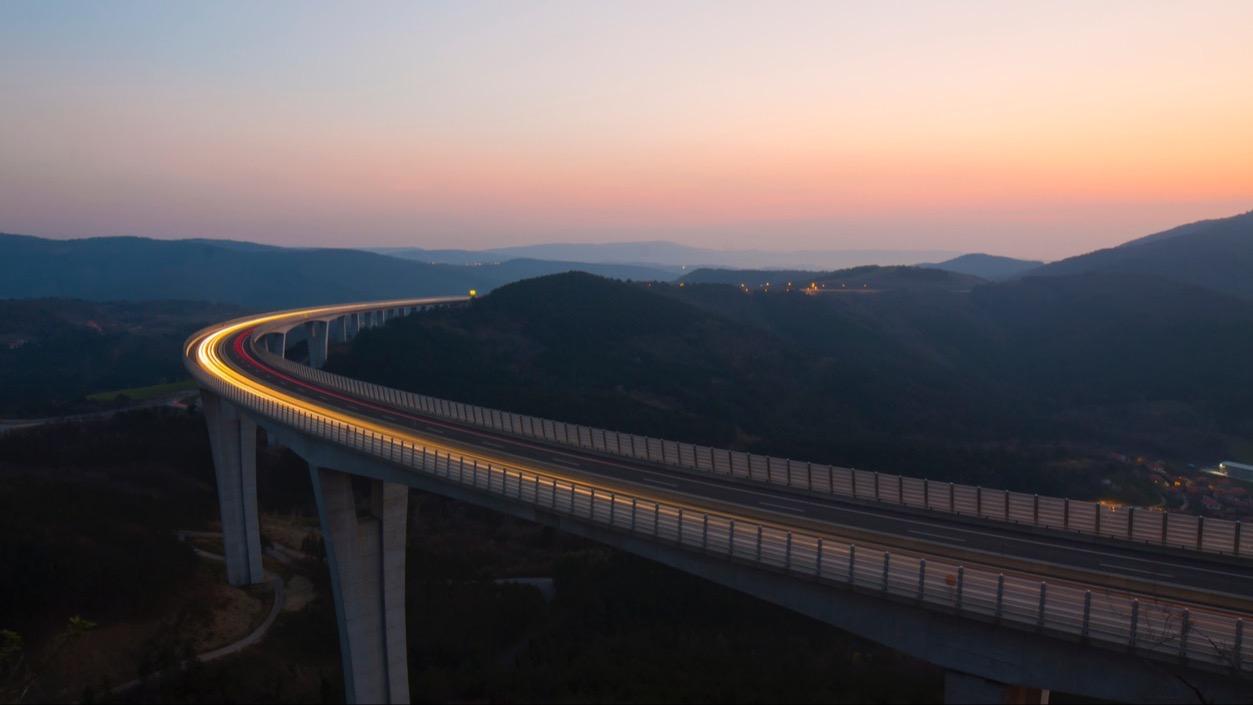
Customer segmentation is the process of categorizing customers into groups with similar traits, needs, or behaviors. This strategy enables companies to create targeted marketing campaigns and personalize their offerings.
Segmentation enhances customer relationships and brand loyalty by aligning messages with specific customer needs.
The following are the most popular types of customer segmentation models that businesses use:
Demographic: Based on age, gender, income, education, etc.
Geographic: Focused on location, climate, or cultural factors
Psychographic: Centered on lifestyle, values, and attitudes
Behavioral: Determined by purchasing habits, brand interactions, and product usage
In this post, we'll explore these (plus many more segmentation models) so you can better understand how to speak to your target audience and get more leads, traffic, and sales.
Key Takeaways:
Customer segmentation is a powerful marketing foundation that groups customers into similar categories for personalized and effective marketing.
When brands segment customers, they increase customer loyalty, reduce churn, and save time crafting new marketing materials.
There are many useful tools to create customer segments, such as machine learning, social listening, and third-party data.
Demographic Segmentation
Demographic segmentation groups customers based on shared characteristics such as age, gender, income, education, and family size. This approach helps businesses target specific customer segments more effectively.
Below are crucial details that define a reliable customer segmentation model.
Age, Gender, and Income
Age segmentation allows companies to tailor products and marketing to different life stages. For example, a skincare brand might offer anti-aging creams to older consumers and acne treatments to teenagers.
Gender-based segmentation can inform product design and advertising strategies (though it's important to avoid stereotypes).
Income segmentation helps businesses price products appropriately and target marketing efforts. Luxury brands often focus on high-income segments, while budget retailers cater to lower-income groups. Companies can use income data to:
Determine pricing strategies
Choose store locations
Develop product features
Education and Family Size
Education level can indicate consumer preferences and buying habits. For example, college graduates may prioritize different products or respond to different marketing messages compared to those with high school diplomas. Family size impacts purchasing decisions across various categories, such as:
Housing needs
Food consumption
Vehicle preferences
Vacation choices
Marketers use family size data to create targeted campaigns. For instance, a minivan manufacturer might focus on households with multiple children. Single-person households, on the other hand, may be more interested in compact living solutions.
By combining these demographic factors, businesses can create detailed customer profiles.
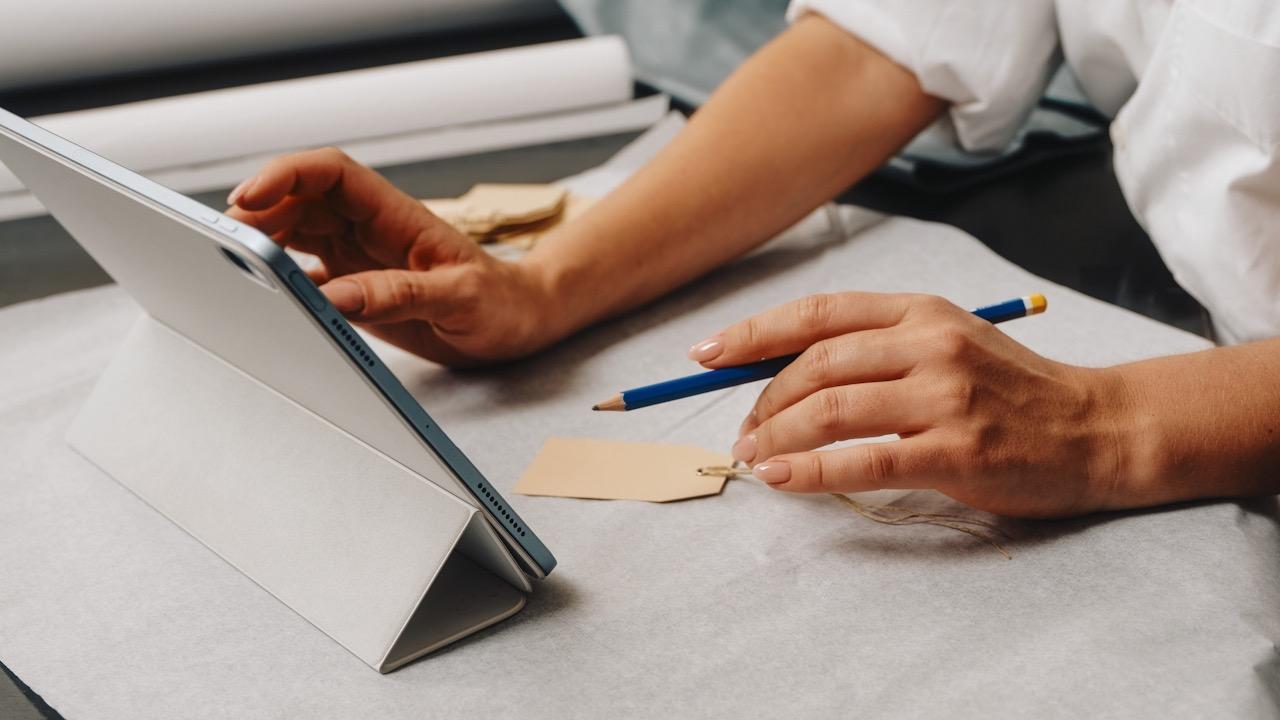
Psychographic Segmentation
Psychographic segmentation groups customers based on psychological attributes like values, attitudes, and lifestyles. This approach helps businesses understand the motivations behind consumer behavior and tailor their marketing strategies accordingly.
Let's take a look at the psychological element of the customer segmentation model.
Lifestyle and Social Status
Lifestyle segmentation examines how people live, spend their time, and allocate their resources. It considers factors such as work-life balance, leisure activities, and consumption patterns. Social status segmentation narrows down a person's position within society, including education level, occupation, and income.
A value-based customer segmentation model can identify loyal customers and areas of lost value. Companies use this information to create targeted marketing campaigns and develop products that align with specific lifestyle preferences.
Luxury brands often focus on high-status segments, offering exclusive products and experiences. In contrast, budget-friendly brands might target price-conscious consumers who prioritize practicality over prestige.
Interests and Activities
This subsection of the customer segmentation model focuses on consumers' hobbies, passions, and recreational pursuits.
Psychographic research uses both qualitative and quantitative data to analyze interests and activities. For example, a survey might ask respondents to describe their morning routine, providing insights into daily habits and preferences.
Sports enthusiasts might be segmented based on their preferred activities, allowing companies to develop specialized equipment or targeted advertising. Tech companies can segment users by their level of interest in new gadgets, tailoring product launches and marketing messages accordingly.
Social media platforms and online behavior provide valuable data for interest-based segmentation.
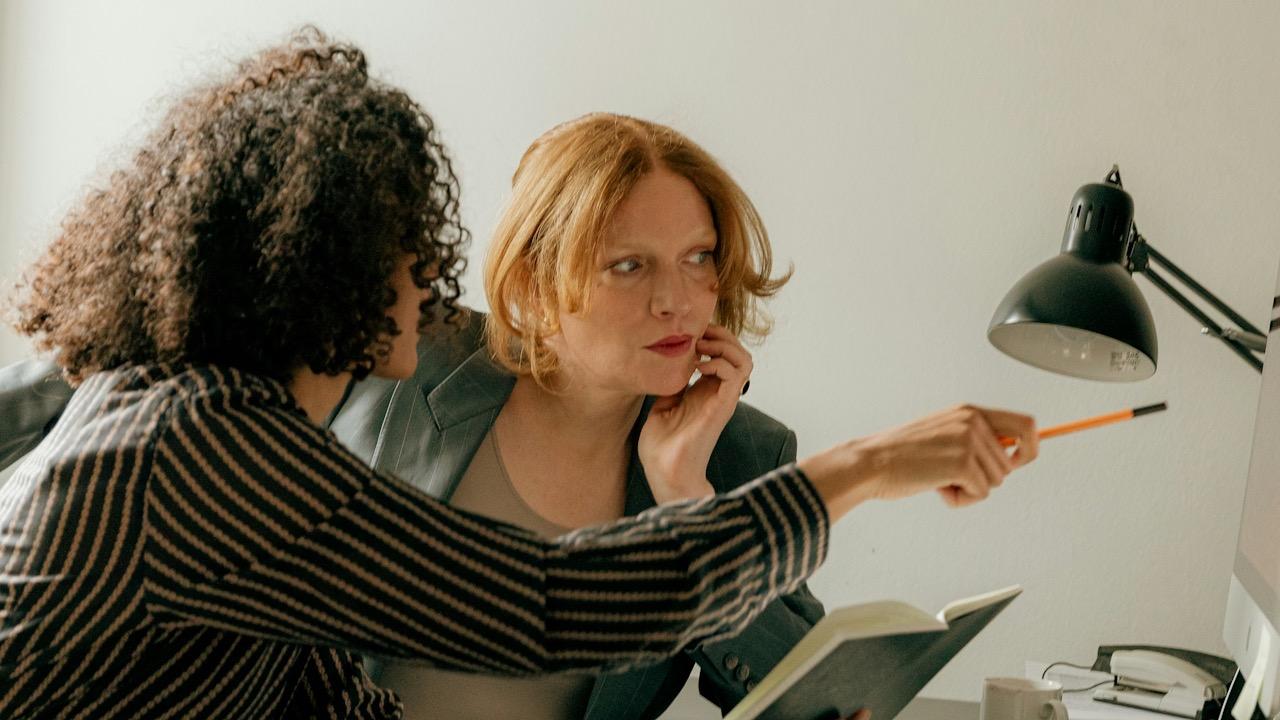
Behavioral Segmentation
Behavioral segmentation groups customers based on their actions, interactions, and purchasing patterns. This customer segmentation model helps businesses understand how customers engage with products or services.
Buying Behaviors and Patterns
Behavioral segmentation examines how customers make purchasing choices. It looks at factors like frequency, timing, and motivation behind purchases.
Businesses can categorize customers as one-time buyers, occasional shoppers, or frequent purchasers. By studying past transactions, companies can predict future buying behavior and identify cross-selling opportunities.
Some customers exhibit brand loyalty, consistently choosing the same product or company. Others may be price-sensitive, always seeking the best deals.
Usage and Engagement
This aspect of behavioral segmentation focuses on how customers use products or services after purchase. It examines factors such as frequency, duration, and intensity of use.
Customer engagement levels can vary widely. Some users might be highly active, while others rarely interact with the product. By tracking these patterns, businesses can identify opportunities to boost engagement and reduce churn.
Usage data helps companies spot potential issues in the customer journey. For example, if many users abandon a product after a specific point, it may indicate a problem.
Behavioral segmentation also helps identify loyal customers who are more likely to make repeat purchases. These customers often provide valuable feedback and can become brand advocates. Understanding what drives their loyalty can help businesses replicate this success with other segments.
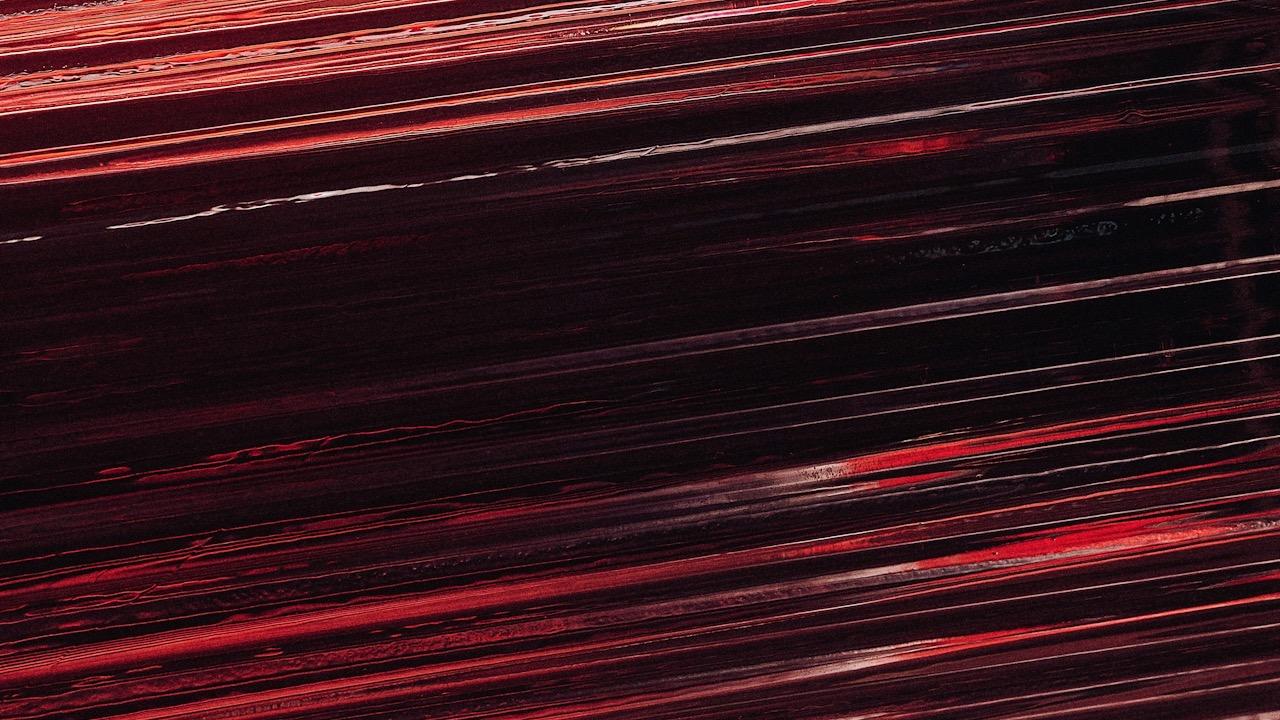
Geographic Segmentation
Geographic segmentation divides customers based on their physical location. This customer segmentation analysis allows businesses to customize strategies for different regions and leverage local market characteristics.
Location-Based Strategies
Geographic segmentation uses data like zip codes, cities, and countries to group customers, then tailor products and marketing to specific areas. For example, a clothing retailer might stock winter coats in northern regions and swimwear in coastal areas.
Climate and population density also influence strategies. Urban areas may require different approaches than rural ones. A food delivery service might offer faster delivery times in densely populated cities.
Local economic conditions affect pricing and product offerings. Higher-income areas may support premium products, while budget-friendly options might perform better in lower-income regions.
Cultural Considerations
Geographic segmentation accounts for cultural differences across regions. Language preferences vary by location, impacting marketing materials and customer support.
Local customs and traditions influence product design and marketing campaigns. A restaurant chain might adapt its menu to include regional specialties or ingredients.
Religious practices in certain areas can affect product demand and marketing timing. For instance, food companies may adjust their offerings during religious holidays.
Consumer behavior patterns differ geographically. Some regions may prefer online shopping, while others value in-store experiences.
AI-Driven Segmentation Models
The AI-powered customer segmentation model has revolutionized how businesses analyze and group their customers. These advanced systems use machine learning algorithms to process vast amounts of data and identify meaningful patterns.
Unlike traditional methods, AI models can handle complex, multi-dimensional data sets. They consider numerous factors simultaneously, including purchasing behavior, demographics, and online interactions.
AI segmentation models offer several key advantages:
Improved accuracy in customer classification
Real-time updates as new data becomes available
Ability to uncover hidden customer segments
AI-driven customer segmentation has become more accessible. Many platforms now offer no-code solutions, allowing businesses of all sizes to leverage these powerful tools.
These models excel at predicting future customer behavior. By analyzing historical data, AI can forecast likely purchases, churn risks, and lifetime value for each segment.
Personalization is another strength of AI segmentation. The models can recommend highly targeted marketing strategies for each identified group.
AI segmentation tools often integrate with existing marketing platforms. This allows for seamless implementation of insights into email campaigns, website personalization, and ad targeting.
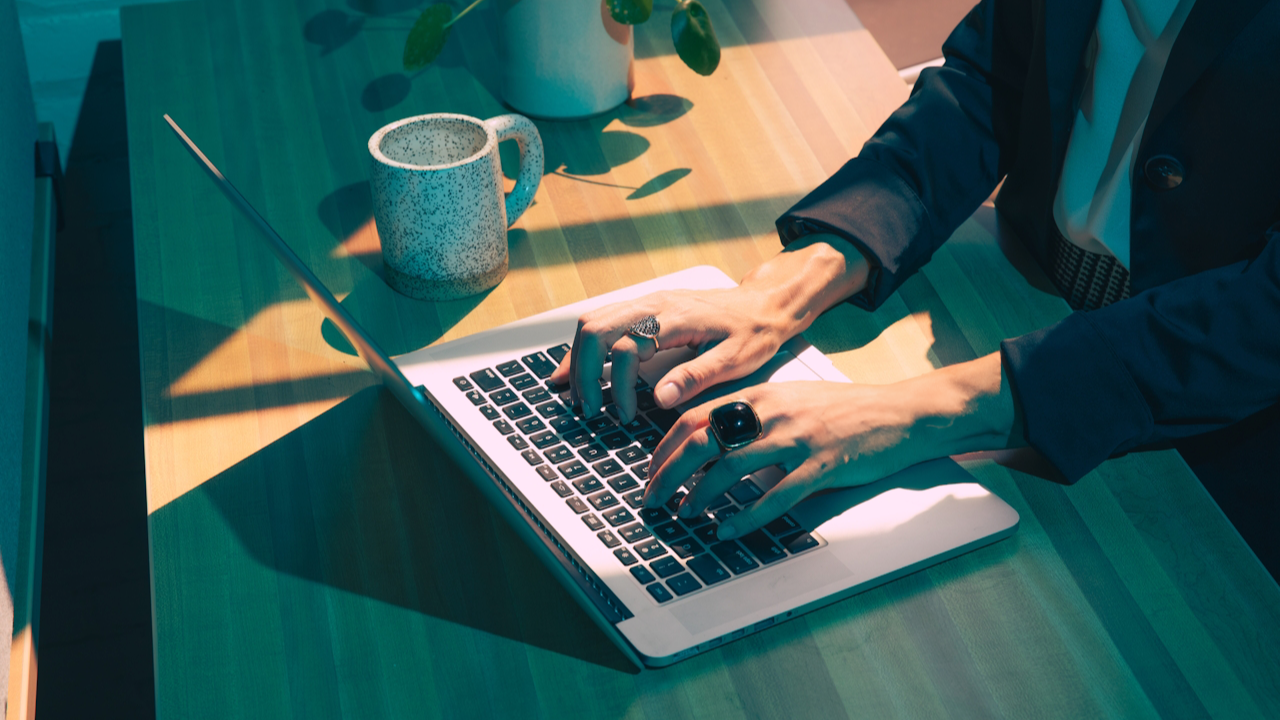
Technographic Segmentation
Technographic segmentation categorizes users based on their technology preferences and usage patterns. This approach helps companies understand how customers interact with digital products and services.
Key factors in technographic segmentation include:
Device types (desktop, mobile, tablet)
Operating systems
Browser choices
Software applications used
By analyzing these elements, businesses can tailor their product features to match user preferences. For example, a company might optimize its mobile app for iOS users if data shows a higher adoption rate among that group.
Technographic data provides insights into:
Technology adoption rates
Digital literacy levels
Preferred communication channels
A tech-savvy segment might respond well to advanced feature promotions, while less tech-oriented users may need simpler messaging.
User behavior analysis is also crucial in technographic segmentation. It reveals how different user groups interact with technology, informing decisions on:
Feature development priorities
User interface design
Technical support resources
Companies can use technographic data to identify opportunities for product improvements and new market entry.
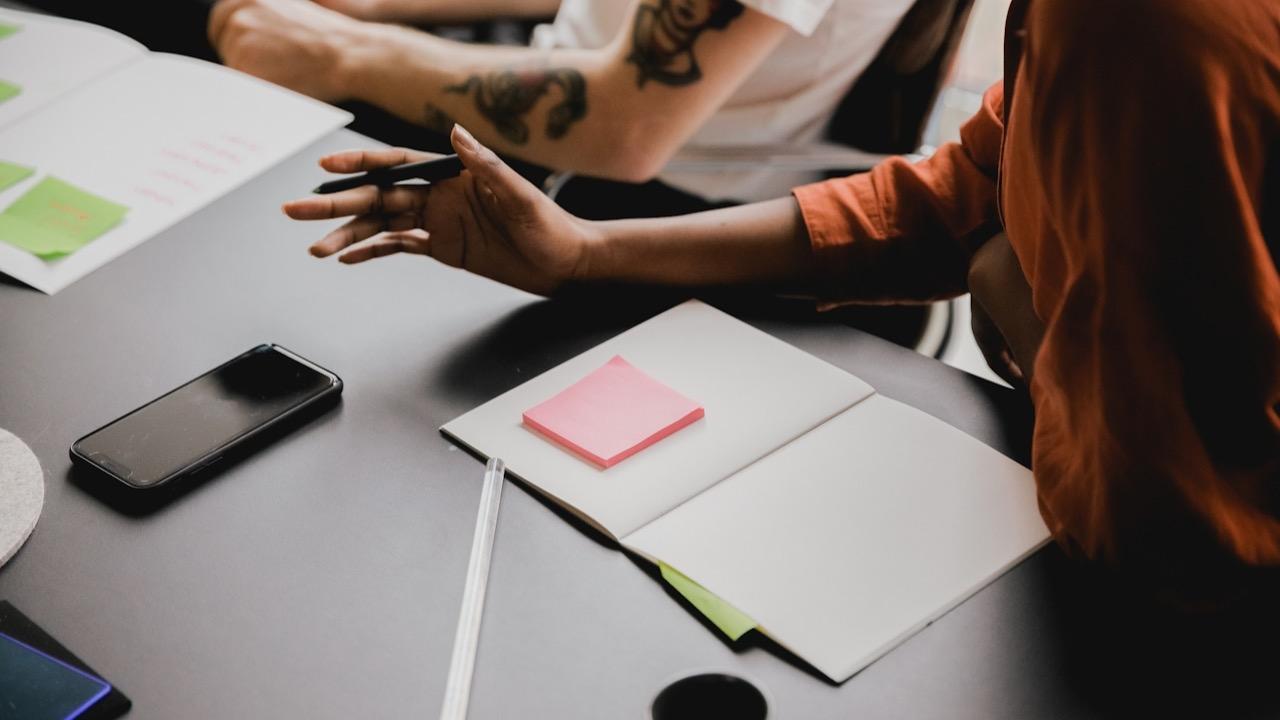
Needs-Based and Value-Based Segmentation
Needs-based and value-based segmentation models group customers according to their requirements and perceived value.
Assessing Customer Requirements
Needs-based customer segmentation categorizes customers by their specific needs, preferences, and pain points. This model helps companies recognize unmet customer needs and develop products or services to address them.
For example, a software company might segment users based on their need for specific features like data analysis, collaboration tools, or mobile access.
To implement needs-based segmentation, you need to:
Conduct customer surveys and interviews
Analyze product usage data
Review customer support inquiries
Identify common pain points and desires
By understanding customer requirements, businesses can create more effective marketing messages and product improvements.
Pricing and Value Propositions
Value-based customer segmentation focuses on the perceived value customers place on a product or service. This approach helps companies determine optimal pricing strategies and develop compelling value propositions for different customer groups.
Key steps in value-based customer segmentation are:
Assessing the customer willingness to pay
Identifying features or benefits most valued by each segment
Developing tiered pricing models or customized packages
For instance, a streaming service might offer different subscription tiers based on video quality, number of simultaneous streams, and ad-free viewing options. This allows them to capture value from price-sensitive and premium customers alike.
Firmographic Segmentation
Firmographic segmentation is a key component of market segmentation for business-to-business (B2B) companies. It divides organizations into groups based on shared characteristics.
Common firmographic criteria include:
Company size
Number of employees
Industry type
Annual revenue
Geographic location
By understanding the unique needs of different company segments, B2B marketers can create a more effective marketing strategy.
Targeted marketing becomes more precise with firmographic data. It allows companies to focus resources on the most promising segments and customize their offerings accordingly.
Benefits of firmographic segmentation include:
Improved lead qualification
More relevant product development
Enhanced customer communication
Increased marketing efficiency
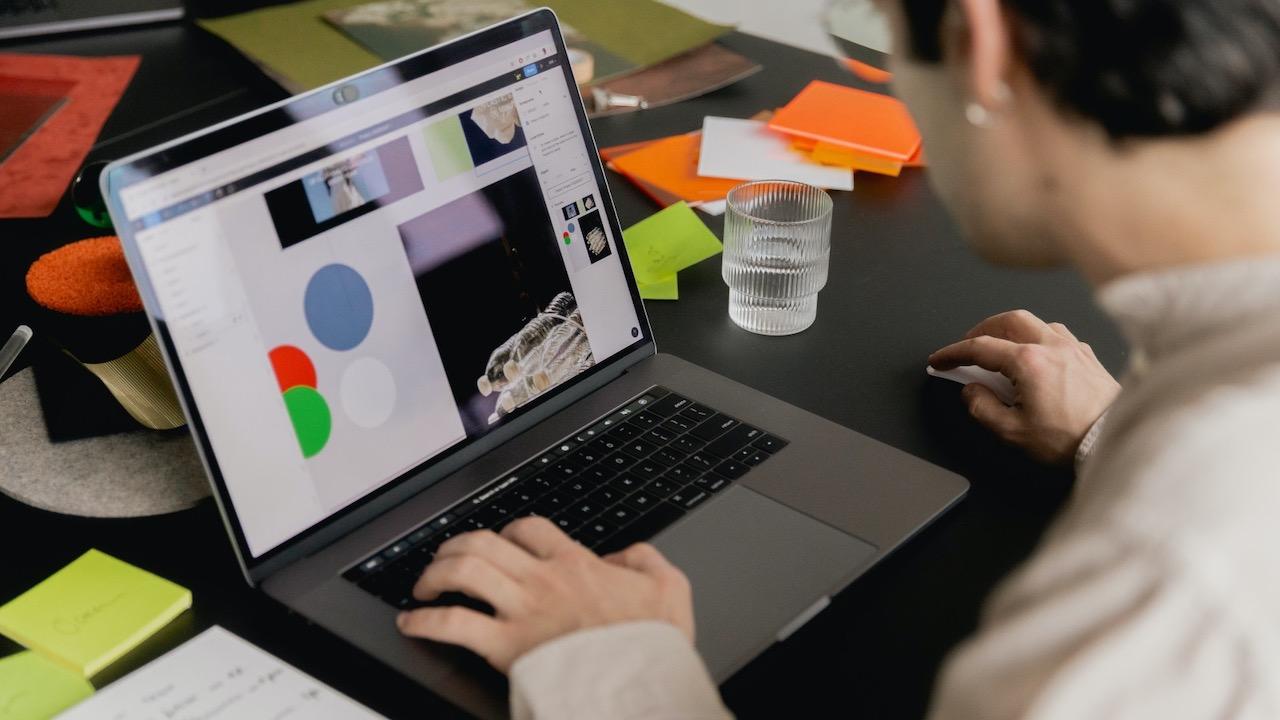
Segmentation Using Machine Learning
Machine learning enables businesses to perform customer segmentation analysis with greater precision. It analyzes large datasets to identify patterns and group customers based on shared characteristics and behaviors.
Predictive Analytics
Machine learning algorithms power predictive analytics for customer segmentation. These tools analyze historical data to forecast future customer actions and preferences. K-means clustering is a popular technique that divides customers into distinct groups based on similarities.
Random forests and decision trees help identify key factors influencing customer behavior. These models can predict which products a customer is likely to purchase next or their potential lifetime value.
Neural networks excel at recognizing complex patterns in customer data. They can uncover hidden relationships between variables that humans might miss, leading to more nuanced customer segmentation.
Model Accuracy and Adaptability
The accuracy of machine learning customer segmentation models depends on data quality and algorithm selection. Regular testing and validation ensure models perform well on new data.
Real-time analysis allows models to update as new information becomes available. This adaptability helps businesses respond quickly to changing customer needs and market trends.
Ensemble methods combine multiple algorithms to improve accuracy.
Continuous monitoring and refinement are crucial. As customer behaviors evolve, so too must a brand's customer segmentation model to maintain their relevance.
Data Sources and Integration for Segmentation
Effective customer segmentation relies on comprehensive data collection and seamless integration across platforms.
Leveraging First-Party, Second-Party, and Third-Party Data
First-party data, collected directly from customers, forms the foundation of customer segmentation efforts. This includes purchase history, website interactions, and customer service records. Its accuracy and relevance make it invaluable for personalization.
Second-party data comes from trusted partners, providing additional context. For example, an airline might share travel preferences with a hotel chain, enriching both companies' customer profiles.
Third-party data from external providers can fill gaps in customer understanding. It may include demographic information, lifestyle data, or broader market trends. While less specific, it can help identify new opportunities and expand reach in a brand's customer segmentation strategy.
Combining these data types creates a 360-degree view of customers, enabling more precise customer segmentation.
Integrating Data from CRMs, Social Media, and IoT Devices
Customer Relationship Management (CRM) systems serve as central hubs for customer data. They store interaction histories, preferences, and purchase patterns, making them crucial for segmentation.
Social media platforms offer insights into customer interests and sentiments. By analyzing social data, companies can refine their segments based on lifestyle choices and brand affinities.
Internet of Things (IoT) devices provide real-time data on product usage and customer behavior. For instance, smart home devices can reveal patterns in energy consumption, informing segmentation for utility companies.
Integrating these diverse data sources allows for more nuanced and accurate customer segmentation. This integration enables businesses to create dynamic segments that update in real-time based on the latest customer interactions and behaviors.
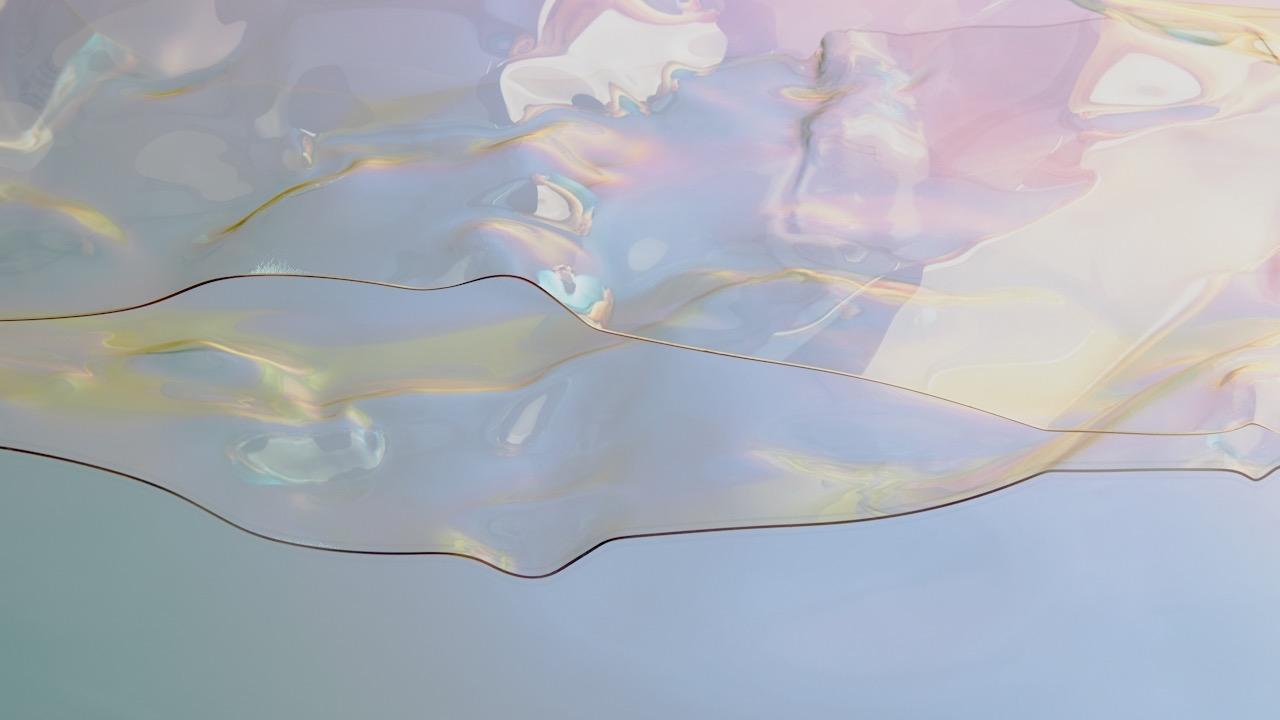
Customer Segmentation Strategies
Effective customer segmentation strategies enable businesses to target specific customer groups with precision. These approaches involve implementing models, then continuously refining segments.
Implementing Segmentation Models
Customer segmentation models form the foundation for successful marketing and sales efforts. To implement these types of customer segmentation, businesses should start by collecting relevant data on their customers. This can include demographic information, purchase history, and behavioral patterns.
Next, companies need to choose the most suitable segmentation criteria. These may be based on factors like geographic location, value, or behavior. For example, a retailer might segment customers by their average order value and purchase frequency.
Once criteria are established, businesses can use data analysis tools to divide their customer base into distinct groups. It's crucial to ensure each segment is large enough to be profitable, yet specific enough to allow for targeted marketing efforts.
Monitoring and Adjusting Segments
Customer segments are not static and require regular evaluation. Businesses should set up systems to track the performance of each segment over time. Key metrics might include conversion rates, customer lifetime value, and response to marketing campaigns.
Regular analysis of these metrics helps identify which segments are performing well and which may need refinement. For instance, if a segment's engagement rates drop, it might signal a need to adjust the targeting strategy or reassess the segment's characteristics.
Companies should also stay attuned to market changes and evolving customer needs. This might involve conducting periodic surveys or focus groups to gather fresh insights. As customer preferences shift, companies must be ready to create new segments or merge existing ones.
Enhancing Customer Experience with Segmentation
Different types of customer segmentation allows businesses to deliver relevant and personalized experiences. By analyzing customer data and behaviors, companies can tailor their offerings and communications to better meet individual needs.
Personalization Techniques
Enhanced customer experiences stem from effective segmentation. Companies can use purchase history and behavioral data to create detailed customer profiles. These profiles enable targeted marketing messages and product recommendations.
Email campaigns can be customized based on segment preferences. For example, a clothing retailer might send different promotions to fashion-forward young adults versus budget-conscious families.
Websites can display personalized content and product suggestions. An e-commerce site could highlight different items for frequent buyers compared to first-time visitors.
Improving Customer Satisfaction
Segmentation helps businesses boost customer satisfaction by addressing specific needs. Customer satisfaction scores often improve when companies tailor their approach to different groups.
Customer service can be optimized for each segment. For example, high-value customers might receive priority support, while self-service options could be prominent for tech-savvy users.
Product development can focus on solving pain points for key segments, such as a software company adding features requested by its enterprise clients.
Pricing strategies can be adjusted to match segment price sensitivity. Luxury brands may offer exclusive perks to their top-tier customers.
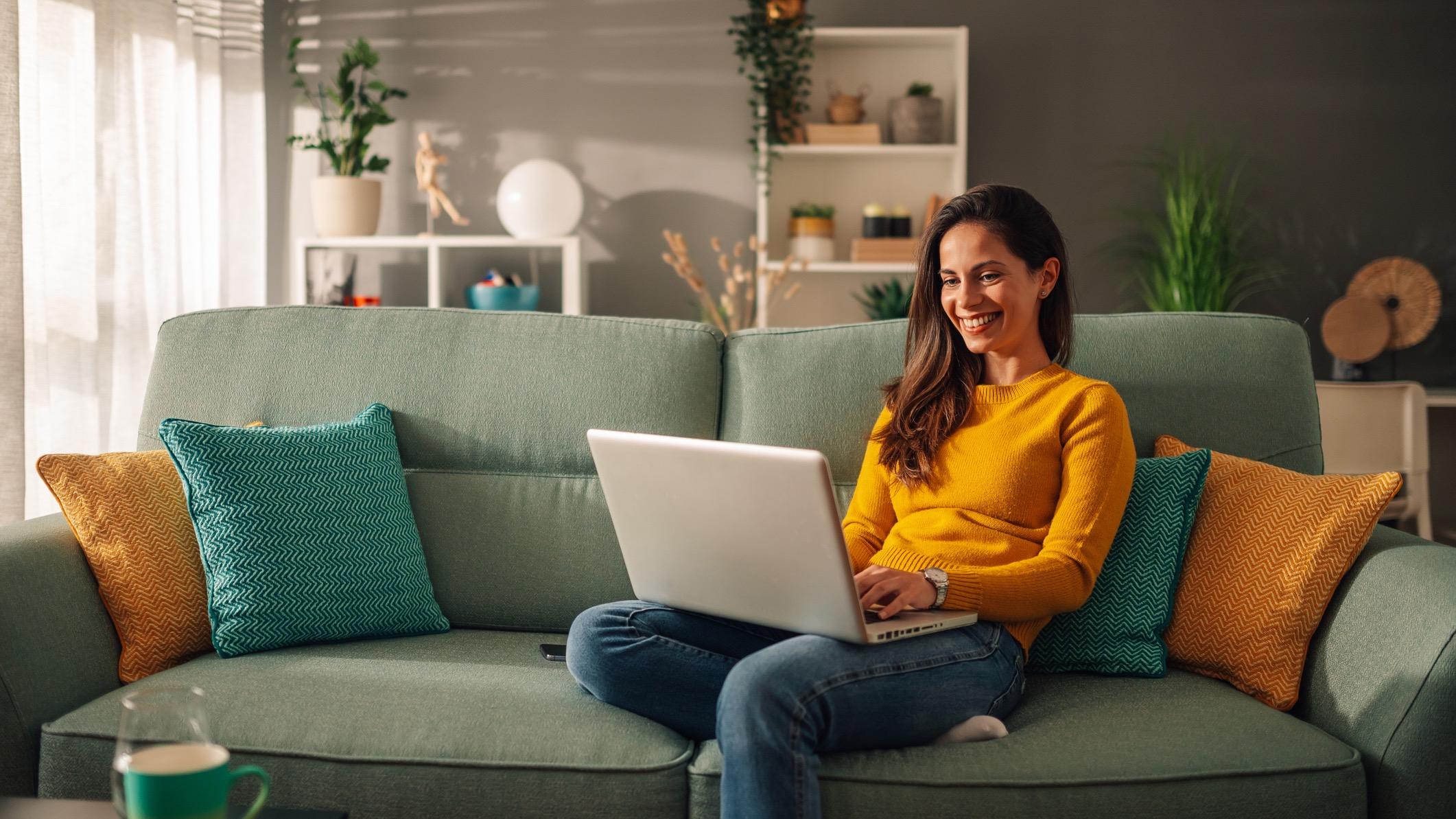
Challenges and Limitations in Implementing Segmentation Models
Implementing customer segmentation models can present several hurdles for businesses. One common issue is data quality and availability. Without accurate and comprehensive customer information, segmentation efforts may yield unreliable results.
Another challenge is choosing the right segmentation criteria. Businesses must carefully select relevant factors that align with their goals and customer base. Incorrect choices can lead to ineffective groupings and wasted resources.
Actionability of segments is crucial. Some models may create segments that are difficult to target or engage with through existing marketing channels.
Technical limitations can also impede implementation. Organizations may lack the necessary tools or expertise to properly analyze data and create meaningful segments for their marketing efforts.
Maintaining up-to-date segmentation is an ongoing challenge. Customer behaviors and preferences change over time, requiring regular updates to keep models relevant. Static segmentation models can become outdated quickly in fast-moving markets.
Lastly, privacy concerns and data protection regulations can limit the types and extent of customer data that can be collected and used for segmentation purposes.
Metrics and Analysis in Segmentation
Segmenting customers effectively requires careful measurement and analysis to ensure strategies are working as intended.
Measuring Segmentation Success
Customer surveys provide valuable insights into segmentation effectiveness. These surveys can gauge customer satisfaction, brand perception, and product preferences across different segments. Net Promoter Score (NPS) is a widely used metric to measure customer loyalty and likelihood to recommend.
Customer service interactions also offer important data points. Response times, resolution rates, and customer feedback scores for each segment can highlight areas for improvement in personalized service delivery.
Retention rates are crucial indicators of segmentation success. Tracking how long customers in each segment stay with the company reveals the effectiveness of targeted retention strategies.
Customer Lifetime Value (CLV) calculations for each segment show which groups generate the most long-term revenue. This metric helps prioritize resources and tailor offerings to high-value segments.
Conversion Rates and Marketing ROI
Conversion rates are key performance indicators for segmentation-based marketing campaigns. Tracking how often leads from different segments become paying customers helps refine targeting and messaging.
Click-through rates and engagement metrics for segment-specific email campaigns or ads indicate the relevance of content to each group. Higher engagement often correlates with better conversion potential.
Cost per acquisition (CPA) broken down by segment reveals which groups are most cost-effective to target. This information guides budget allocation across marketing channels.
Return on investment (ROI) calculations for segment-specific marketing initiatives show which efforts generate the best results.
Frequently Asked Questions
What are the key approaches to segmenting customer groups within a market?
Customer segmentation models typically include demographic, psychographic, behavioral, and geographic approaches. Demographic segmentation focuses on age, gender, income, and education.
Psychographic segmentation considers lifestyle, values, and attitudes. Behavioral segmentation looks at purchasing habits and product usage. Geographic segmentation groups customers by location.
How can machine learning algorithms be applied to customer segmentation?
Machine learning algorithms can analyze large datasets to identify patterns and groupings that humans might miss. These algorithms can process customer data to create more precise and dynamic segments.
What is the difference between demographic and psychographic segmentation?
Demographic segmentation uses measurable characteristics like age, gender, and income. Psychographic segmentation examines less tangible factors such as personality traits, values, and lifestyles. Psychographic data often provides deeper insights into customer motivations and preferences.
In what ways can customer segmentation enhance marketing efficiency?
Customer segmentation allows marketing teams to create targeted campaigns for specific groups. This approach can lead to higher conversion rates and better return on investment. It also helps in developing personalized product offerings and communication strategies.
How do B2B customer segmentation models differ from B2C models?
B2B segmentation often focuses on company size, industry type, and decision-making processes. B2C models typically emphasize individual consumer characteristics and behaviors. B2B segments may be smaller and more specialized, while B2C segments can be broader and more numerous.
What role does data science play in developing effective customer segmentation models?
Data science helps in creating more sophisticated segmentation models that can adapt to changing customer behaviors. Data scientists use statistical techniques and machine learning to refine and validate segmentation approaches.
What are the 4 P's of marketing segmentation?
The 4 P's of marketing segmentation are Product, Price, Place, and Promotion. These elements help tailor marketing strategies to different customer segments.
Create Data-Driven Customer Segmentation Models With Rightpoint
From grouping existing customers to ushering in new customers, you need data for your segmentation models. At Rightpoint, we provide customer research, analytics, and insight to keep your marketing strategies relevant.
Are you ready to start encouraging repeat customers with tailored and personalized messaging? Contact us today to start refining your segmentation model for results that last.